Learn how to calculate a confidence interval and gain a solid understanding of statistical certainty quickly and easily.
Ever felt like statistics is speaking a foreign language? Buckle up! We’re about to demystify confidence intervals, making you the data whisperer you were destined to be.
From understanding the basics of estimating population parameters to mastering the calculation of mean and standard deviation, we’ve got it all covered.
Ready to dive in and turn those baffling intervals into crystal-clear insights? Welcome aboard, math wizard!
Key takeaways:
- Confidence intervals estimate population parameters from sample data.
- The wider the interval, the less precise the estimate.
- Confidence level expresses certainty in capturing the true parameter.
- Choose between one-sided and two-sided intervals based on research question.
- Calculate the mean, standard deviation, and use the formula to find the confidence interval.
What Is a Confidence Interval?
Imagine you’re baking cookies, and your Grandma wants to know how many chocolate chips are in one cookie, on average. Instead of counting every chip in every cookie (because, really, who has time for that?), you take a few samples and make an estimate. That’s your average. Now, you want to tell Grandma how confident you are in that estimate.
Enter the confidence interval. It’s a range that likely contains the true average number of chips, based on your sample. Think of it as a safety net around your average, saying, “I’m pretty sure the real number falls somewhere in here.”
- Points to get the gist:
- It’s created from your sample data to estimate a population parameter.
- It tells you the range where the true value is likely to be.
- The wider the interval, the less precise your estimate.
Plus, the more samples you take, the narrower and more accurate your interval becomes. It’s like upgrading from a safety net to a laser-focused chocolate chip detector!
What Is a Confidence Level?
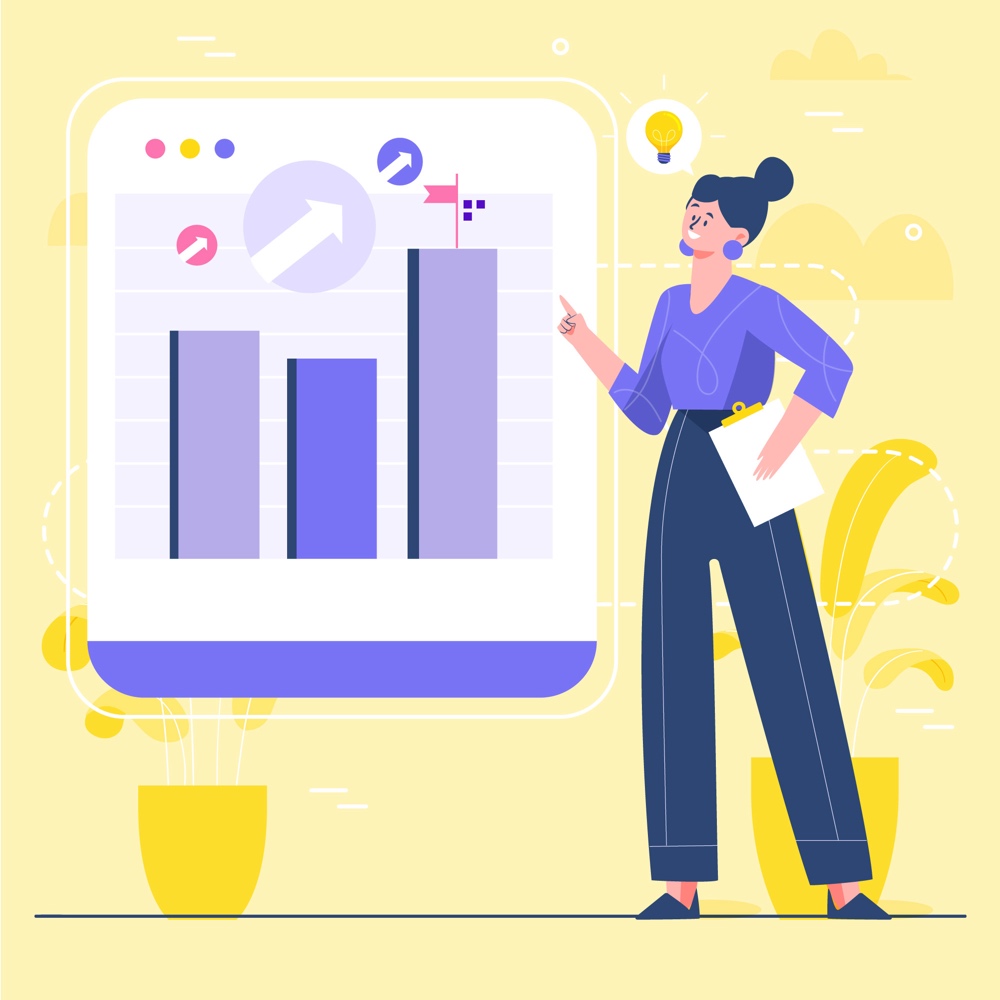
Alright, let’s dive into this. Picture a confidence level as your trusty, reliable friend who’s got your back 95% of the time—or whatever percentage you choose. It’s a way to express how certain you are that your confidence interval captures the true population parameter.
- Confidence Level Percentage: Usually, people go for the 95% comfort zone because it’s like that perfect, snug blanket—highly reliable without being overbearing. But hey, you could also go for the 99% if you’re feeling extra cautious or 90% if you’re more of a risk-taker.
- Margin for Error: The confidence level directly influences the margin of error. Higher confidence level? Bigger margin. Lower confidence level? Smaller margin. It’s a balancing act, like deciding between a large safety net or a tightrope walk.
- Statistical Zing: It’s tied to z-scores or t-scores, depending on whether you’re using a normal distribution or t-distribution. Think of these as the bells and whistles that adjust how wide or narrow your interval gets.
Imagine yourself playing board games with friends, and you have varying degrees of confidence in their poker faces. Your confidence level is akin to your gut feeling about whether they’re bluffing but backed up with statistical swagger. There you have it—confidence level in a nutshell. Simple, right?
One-Sided Confidence Intervals Vs. Two-Sided Confidence Intervals
A pivotal step in confidence intervals is deciding between one-sided and two-sided intervals. This choice hinges on your specific research question.
One-sided confidence intervals predict a range in only one direction. Imagine guessing the maximum speed of a cheetah, you’re hunting for an upper limit. Use a one-sided interval to claim, “The cheetah can run no slower than 75 mph.”
Two-sided intervals cover uncertainties in both directions, offering a range you believe includes the true mean. Think of estimating average daily coffee consumption: aiming to stay within reasonable highs and lows. A two-sided interval might claim, “People drink between 2 and 4 cups a day.”
Here’s the twist: two-sided intervals are more common because they reflect general uncertainty snuggly.
Keep this tidbit in your statistical toolkit: use one-sided for directional predictions, two-sided for general guesses.
Step #1: Find the Number of Samples (n)
First things first, count your blessings—or in this case, your samples. The number of samples you have denoted by ‘n’ is fundamental. Think of it as headcount at a party; the more the merrier.
Why is it crucial? Because the quantity impacts the reliability of your results. Greater sample sizes typically yield more accurate estimates. Sample size helps in reducing the margin of error. Fewer samples mean more guesswork; more samples mean less blindfolded dart throwing.
Counting is straight-forward: if you’ve got 50 test results, well, n=50. But remember, quality over quantity is also important. Ensure your samples are representative of the population. No cherry-picking! Unless we’re talking about actual cherries, then please, go ahead.
Step #2: Calculate the Mean (x) of the Samples
To figure out your sample mean, take the sum of all your sample values and then divide by the number of samples. Think of it as throwing a party and counting how many slices of pizza everyone ate.
Summing up all the pizzas devoured gives you the total number. Divide that by how many friends attended, and voilà—you have the average.
Here’s how you do it in practice:
- Add up all the sample values.
- Divide that total by the number of samples.
For example, if you sampled five people and their values were 2, 4, 6, 8, and 10, the sum is 30. Divide by 5 and you get 6. Easy peasy, right?
Remember, the mean is the anchor of your confidence interval, much like that pizza is the anchor of your party. Without it, everything falls apart.
Step #3: Calculate the Standard Deviation (s)
Now, here’s where things get a bit thrilling—calculating the standard deviation! Think of it as measuring the “spread” of your data points. To do this, follow these steps and avoid the melodrama:
First, find the mean of your data set. If your data set represents shoe sizes, the mean is simply the average shoe size. Easy-peasy, right?
Next, subtract the mean from each data point to find the “deviation” for each. These deviations show just how far off each shoe size is from average—because no one’s perfectly average, after all!
Now, square each deviation. Why? Squaring gets rid of pesky negative values and gives added weight to larger differences. Squared deviations are just deviations with a bit of muscle.
Then, sum up all those squared deviations. This total helps us understand the overall variability in your data.
Finally, divide this sum by the number of data points minus one. This gives you the “variance.” But wait, there’s more! Take the square root of this variance, and voila—you’ve got your standard deviation. It’s like finding the root of all chaos in your data set.
With that, you’ve measured the spread of your data, bringing you one step closer to the thrilling world of confidence intervals.
Step #4: Decide the Confidence Interval That Will Be Used
Alright, you’ve gathered your data and crunched some numbers. Now, let’s talk confidence. Essentially, deciding on your confidence level is choosing how sure you want to be about your interval containing the true population parameter.
Typically, folks go for a 95% confidence level. Why? It’s a good balance between being confident and not overly conservative. It’s like aiming for a B+ in life – good enough to win, but not too paranoid.
If you’re feeling really cautious, there’s the 99% confidence level, the equivalent of wrapping yourself in bubble wrap before a walk in the park.
For the risk-takers, the 90% level is like going out in the rain without an umbrella – you’re tempting fate, but hey, sometimes you want to live on the edge.
Just remember, higher confidence means a wider interval, because you’re casting a bigger net to catch the true mean. While a lower confidence level means a narrower interval, but you might miss the mark.
Choose wisely, because these percentages resonate in the mathematical voodoo we do next.
Step #5: Calculate the Following Formula
Here’s where the rubber meets the road, folks. We’ve got numbers, we’ve got means, and we’ve got standard deviations. Let’s plug them in and get our confidence interval. The magic formula is:
x ± Z * (s/√n)
Hold onto your hats, here’s how it breaks down:
- x is the mean (a.k.a the star of the show)
- Z is the Z-value (think of it as the confidence level’s secret sauce)
- s is the standard deviation (the spread’s partner-in-crime)
- n is the number of samples (the more, the merrier)
Imagine Z as the bodyguard of our mean. For a 95% confidence level, Z usually stands at 1.96, like a muscled-up bouncer keeping things in check.
In essence, you’re adding and subtracting the product of Z and (s divided by the square root of n) from the mean. This dance produces an interval that tells you, with 95% confidence, where the true mean likely hangs out. Like a party where you’re 95% sure everyone’s in the kitchen.
Keep it simple: plug and chug. You’ll be a confidence interval whiz in no time.
Confidence Interval for Proportions
For this type, grab your magnifying glass because we’re zooming in on proportions. Imagine you want to know the proportion of people who prefer pineapple on pizza (let’s not get into that debate here). Here’s how you calculate:
First, count the number of successes (those brave souls who love pineapple) and the total number of trials (everyone surveyed). Say, 325 out of 500 people are team pineapple.
Next, calculate the sample proportion (p̂) by dividing the number of successes by the total number of trials. So, 325 divided by 500 equals 0.65. Boom, that’s your sample proportion.
For large samples, use the Z-distribution for your critical value (find it on a Z-table or use a fancy calculator if you wish). For a 95% confidence level, that’s 1.96. No, it’s not a secret code, it’s your Z-score.
Then, calculate the standard error (SE). Easy-peasy formula: SE equals the square root of (p̂ times (1 minus p̂) divided by the sample size). In numbers, it’s the square root of 0.65 times 0.35 divided by 500.
Finally, slap that into the formula: p̂ plus or minus (Z-score times SE). This gives you a range in which the true proportion likely falls. Congratulations, you just mathematically justified everyone’s pizza choices. Sort of.
The 95% Confidence Interval Explained
Picture this: You’re throwing darts, aiming for the bullseye. The 95% confidence interval means that 95 out of 100 darts will land within a specific range on the dartboard. Just replace darts with sample data points, and the dartboard range with your calculated interval.
Here’s the scoop:
- Probability Power: This 95% figure is all about the probability — we’d capture the true population mean in 95 out of 100 similar scenarios.
- Margin for Error: The broader your interval, the more confident you are that you’ve captured that elusive population mean. It’s like casting a wide net in the ocean; more fish, but also more seaweed.
- Z-Score Zest: To find your interval, you’ll often use a z-score of 1.96 for that magical 95% confidence. Think of it as your trusty sidekick in this stats adventure.
Essentially, it’s like giving your data a cozy bracket, saying, “Hey, I’m pretty darn sure the real average lies somewhere in here, give or take a bit.” And voilà, confidence in numbers.
Fun fact: it’s not about being 95% sure of your data, it’s about knowing how often this method will hit the mark in the long run. Now, go out there and dart with confidence!