Calculate degrees of freedom by subtracting one from your sample size, or keep reading to understand its broader applications and nuances.
Ever felt like the puppet master of your own data, pulling strings and making statistical magic happen? Well, calculating degrees of freedom is like knowing exactly how many strings you’ve got to work with.
From simple samples to dazzling ANOVAs and chi-square mind-bogglers, this guide’s got all the tricks up its sleeve. Dive in, because mastering degrees of freedom could be your new statistical superpower!
Key takeaways:
- Degrees of freedom represent the number of ways you can manipulate data within statistical rules.
- For a single sample, degrees of freedom are the number of data points minus one.
- In a chi-square test, degrees of freedom are calculated by subtracting one from the number of categories or by multiplying the number of rows minus one by the number of columns minus one.
- In ANOVA, between-group degrees of freedom are calculated as the number of groups minus one, while within-group degrees of freedom are calculated as the total number of observations minus the number of groups.
- For a two-sample t-test, degrees of freedom are calculated by adding the sample sizes of both groups and subtracting two.
What Are Degrees of Freedom?
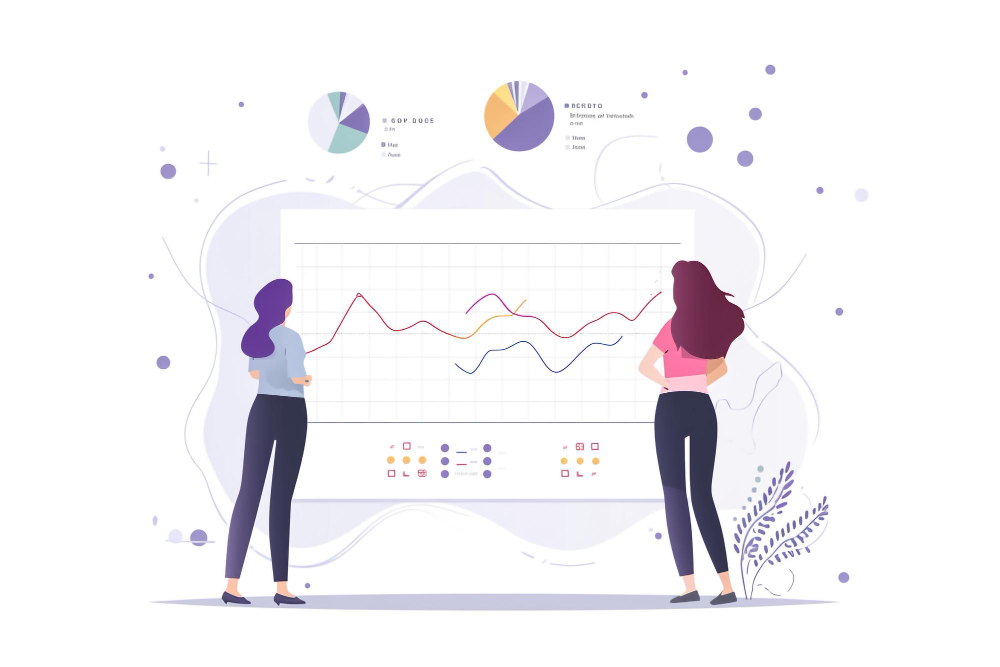
Essentially, think of degrees of freedom (DF) as the number of ways you can wiggle around with your data points while still staying inside the rules of your statistical test.
Imagine you’re tossing a Frisbee with your dog in a field. If you’ve got five data points, it’s like having five Frisbees. Your degrees of freedom are how many throws you’ve got before you start seeing repetitive patterns in your dog’s catch.
For a single sample, degrees of freedom are usually just the number of data points minus one. Let’s say you’ve got 10 test scores. Your degrees of freedom would be 9. Why one less? Because one of those scores is just hanging around to keep everything balanced, like the designated driver at a party.
In essence, DF can change depending on what kind of statistical hullabaloo you’re performing. And yes, the more data points you can play with, the more accurate your test results will be. Got it? Good! Now let’s move on before the data police show up.
How to Find Degrees of Freedom – Formulas
Alright, let’s get down to the nitty-gritty of it. When dealing with degrees of freedom, you’ll mostly be reaching for a handful of formulas. Here are some common scenarios to make it snappy:
- For a single sample t-test, you’re working with: n – 1
- This formula is as simple as it sounds. Take the number of observations (n), subtract one, and voilà, that’s your degrees of freedom. Think of it as removing one for the unseen statistical puppet master pulling the strings.
- With a chi-square test, it’s typically: (number of rows – 1) (number of columns – 1)
- Imagine a grid like a board game. It’s not enough just to survive; you need to account for the complexity by subtracting one from both rows and columns and then multiplying them. Ta-da.
- Now, for one-way ANOVA, you’ll encounter two facets:
- Between groups: k – 1 (k is the number of groups)
- Within groups: N – k (N is the total number of observations across all groups)
- It’s like choosing the best pie slice. First, subtract one from the number of pies (groups) and then consider the leftover pieces after accounting for each group.
- Lastly, we’ll peek at the two-sample t-test: n1 + n2 – 2
- Here, you sum up the sample sizes of both groups and then subtract two. It’s like planning a dinner party but always saving two seats for uninvited surprise guests who never show up.
Now, you’re fully equipped to tackle degrees of freedom with these basic formulas!
Degrees of Freedom for T-test
Alright, let’s demystify the magic numbers behind the t-test!
First, it’s key to know that degrees of freedom (DF) are like the number of chairs at the statistics party – they determine how roomy your analysis can be. For a single-sample t-test, the calculation is straightforward:
DF = n – 1, where n is the number of observations in your sample. Simple math, right?
Now, for a two-sample t-test, things get a tiny bit trickier but not by much. You’ll add together the number of observations in both samples and subtract 2 (DF = n1 + n2 – 2). It’s like letting two friends bring someone to your stat-party but making sure they leave room to wiggle.
Why subtracting? Think of it as accounting for the freedom lost due to using sample data to estimate the mean. It’s like borrowing a cup of sugar and owing your neighbor a favor. Every estimate reduces freedom a bit.
There’s your t-test degrees of freedom! Neat, huh?
Degrees of Freedom for Chi-square
Calculating degrees of freedom for a chi-square test is simpler than finding Waldo in a crowd of Waldos. Here’s the lowdown.
First, consider the categories you’re dealing with. Think of it like sorting socks: blue, red, and the lone pink one. The magic formula is the number of categories minus one. Got four categories? Degrees of freedom equals three. Simple.
Now, if you’re working with a contingency table (fancy talk for a table comparing two variables), the plot thickens, but only a tad. You multiply the number of rows minus one by the number of columns minus one. Rows times columns minus one minus one equals your degrees of freedom. Easy peasy, right?
Why does this matter? Degrees of freedom help you figure out if what you’re seeing is due to random chance or if there’s something fishy going on. So next time someone talks chi-square, you’re all set to dazzle.
Degrees of Freedom for ANOVA
Moving on to ANOVA, degrees of freedom add a sprinkle of complexity, but they’re essential for slicing through the data jungle. Think of them as your trusty machete. Here’s how it works:
Between-Group DF: This measures variation among group means. Calculate it with the formula k-1, where ‘k’ represents the number of groups you’re comparing. If you have 4 groups, your between-group degrees of freedom will be 3. It’s that simple.
Within-Group DF: This sums up the variation within each group and gives you a peek at the data’s personality. Use the formula N-k, where ‘N’ is the total number of observations and ‘k’ is the number of groups. Have 30 samples and 3 groups? You’re looking at 27 within-group degrees of freedom. Easy peasy.
Total DF: Just the missing puzzle piece! It’s found by subtracting 1 from the total number of observations, (N-1). For 40 observations, you get 39.
These bits of statistical sorcery help untangle whether the differences among group means happened by chance or if you’re onto something big. Voilà! You’re now an ANOVA degree of freedom wizard.
Degrees of Freedom for Two-sample T-test
Picture this: two groups, two sets of data, and one burning question – are their means different? Enter the two-sample t-test, our trusty detective in the world of statistics. Now, degrees of freedom here are a tad more intricate. Let’s break it down like a dance move.
First, grab the sample sizes of both groups, n1 and n2. Then, remember their high school reunion – these two need some catching up. Calculate the degrees of freedom by adding their sizes together and subtracting 2: (n1 – 1) + (n2 – 1). Yes, it’s that simple.
Why subtract 2? Humans love complexity, but in essence, each group has one less degree of freedom because we’re using their sample means as estimates. Think of it like those pesky school rules: lose two degrees because you can’t use those means twice.
Still with me? Great. Picture comparing playlists – each song (data point) contributes, but two of them are just there to set the mood (means), hence the drop by 2 in freedom. It’s a statistical spring clean!
And hey, if that math sounds like your Sunday nightmare, many fancy calculators and software will happily do the legwork. Keep it cool and let technology crunch those numbers. You’re not alone in this detective work.
Why Subtract 1 From the Number of Items?
Ah, the enigma of subtracting 1. It’s like the plot twist in a good movie that leaves you scratching your head and saying, “Wait, what?”
Here’s why it happens. When you calculate the variance or standard deviation, you’re estimating the population parameters using your sample data. The first data point is free to be any value. But each subsequent data point constrains the sum of squares to match the calculated mean.
Imagine you have three marbles, each representing an observed value. You count the total number but keep one marble hidden. Now, if you knew two out of three values, the third one isn’t really a mystery anymore. It’s a prisoner to the total.
By removing 1 degree of freedom, you compensate for the fact that the sample mean is used as a point of reference. This adjustment prevents the underestimation of variability.
Think of it as the price you pay for peeking at one of the answers before the quiz is over. Yes, it’s a math game of hide-and-seek. And now, you’re in the know. Enjoy the wisdom!
Degrees of Freedom Calculator
Need a shortcut? Degrees of freedom calculators are the trusty sidekicks you never knew you needed. Plug in your numbers and voilà, the calculator does its magic. But hey, it’s not just about the result; let’s understand a bit behind the curtain:
Think of these calculators as super-smart tools programmed to handle your data’s messy realities. Enter your sample size and any other necessary stats.
They use specific formulas depending on your test – be it t-tests, ANOVA, or chi-square.
Mathematical tricks include subtracting 1 or more from your sample size, based on the type of analysis you’re running.
Instantly translate complex equations into simple, digestible numbers. They save time and reduce human error, which, let’s face it, can be as dependable as a chocolate teapot.
So next time you’re crunching numbers and feeling lost, summon your degrees of freedom calculator and let it do the heavy lifting!
Why Do Critical Values Decrease While DF Increase?
As the number of degrees of freedom (DF) increases, critical values decrease. This happens because with more data points or parameters, our estimates get more specific and closer to the actual values. In other words, we have a clearer picture!
- Larger sample size: Imagine having a thousand people taste-test a new ice cream flavor versus just ten. More data makes our results more reliable.
- Reduced variability: More degrees of freedom mean less room for wild variations. Our results get sharper with a larger dataset.
- Tighter distribution: The spread of your data gets narrower as DF increase, making it less likely to stray far from the average.
Think of it this way: more information means less guesswork and finer adjustments, leading to smaller critical values. More clarity, fewer bumps!
History of Degrees of Freedom
In the annals of statistical lore, the notion of degrees of freedom is like that quirky uncle we all have—useful, a bit mysterious, and with more interesting backstories than we remember to ask about. The concept originated in the early 19th century, thanks to our math buffs: Carl Friedrich Gauss and Pierre-Simon Laplace. They didn’t just invent this term to make our homework harder; they were dealing with the complexities of error analysis and fitting curves to data.
Fast forward a bit to William Sealy Gosset, the brain behind the t-test, who worked for Guinness—yes, the beer company. Guinness didn’t want competitors to learn its secrets, so Gosset published under the pseudonym “Student.” Gosset showed that life gets more complicated when working with smaller sample sizes, and voilà, the degrees of freedom became essential to his calculations.
Sir Ronald A. Fisher, another statistical rock star, further refined the concept in his groundbreaking work in the early 20th century. He made degrees of freedom a staple in various statistical tests, like ANOVA. Fisher’s approach helped researchers understand the variability in their data and created more accurate models.
So, remember, the journey of degrees of freedom from Gauss’s initial ideas to Fisher’s expansions is a testament to mathematical ingenuity and quite the collaborative global effort of historical math whizzes.